Lessons from a Successful Implementation
Transforming Search Accuracy and Customer Satisfaction
In today’s digital age, a seamless customer experience can often mean the difference between a loyal client and a lost opportunity. This is especially true in case of B2B interactions where the quality of such experiences and transactions defines the relationships between businesses and their customers. For us at USEReady, helping reconfigure such interactions, simplifying them and making them more effective – both for organizations and their customers – is our raison d’etre.
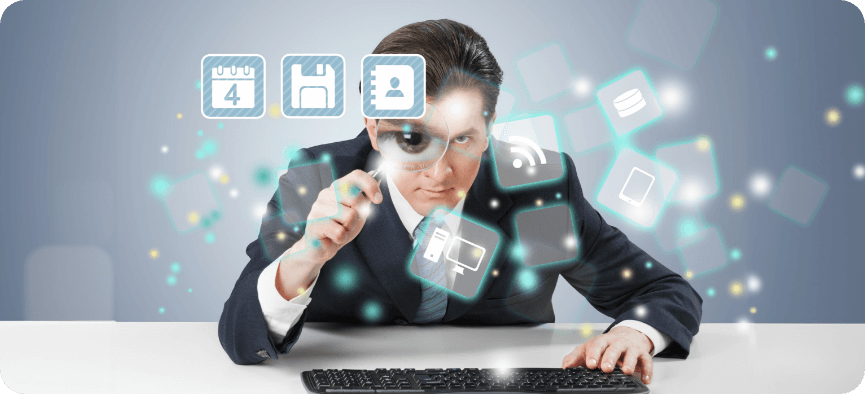
A recent customer success story wherein we transformed the product search journey for one of the world’s leading manufacturers of specialty materials, using the power of Generative AI (GenAI), exemplifies this mission of ours.
Introduction to Generative AI in Product Search
Key Strategies for AI Implementation
Impact of Generative AI on Search Performance
Insights from a Real-World Success Story
Understanding Generative AI
Steps to Implement AI in Product Search
Measuring Success and Impact
Future Trends in AI-Driven Search
Our client, a Fortune 500 firm manufactured specialty materials and chemicals for a diverse range of industries. While their commitment to innovation and quality of their products had always been top-notch, their earlier keyword-based search system for their customer website didn’t always offer the right experience. Customers often struggled to find the specific products they needed because the system relied on exact keyword matches. This frustration hindered their ability to make informed decisions and ultimately impacted their business.
USEReady’s Decision Intelligence team knew there had to be a better way. We implemented a comprehensive solution that replaced the outdated keyword search with a sophisticated Natural Language Processing (NLP) engine. Here’s how we did it:
Building a Knowledge Base
Imagine a giant library filled with information about every product the client offers. We harnessed data from various sources, like Snowflake, Word documents, and Excel spreadsheets, to create this comprehensive product knowledge base. It included detailed features, properties, and real-world applications for each product.
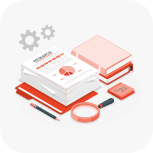
Understanding the Customer Language
People don’t search for products using robotic keywords. They use natural language! Therefore. We built a custom NER model trained on industry-specific data. This model acts like a super translator, identifying key terms like features, properties, and applications within a customer’s search query.
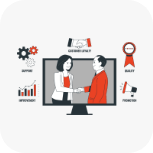
Finding the Perfect Match
We combined two powerful search techniques: lexical and semantic search. Lexical search works like a traditional library card catalog, matching keywords and phrases with the product knowledge base. Semantic search goes a step further – it considers the meaning and intent behind a search query to deliver the most relevant results, even if the exact keywords aren’t used.
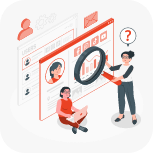
Personalized Recommendations with a Human Touch
We incorporated OpenAI’s GenAI platform to generate natural language responses and product recommendations based on the search results. By the way, this personalized approach goes beyond just showing products; it suggests alternatives and explores similar options, empowering customers to make well-informed decisions.
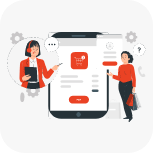
When deployed, the GenAI-powered search solution delivered impressive results almost immediately with customers embracing the intuitive, natural language search interface, ditching the frustration of keyword-based searches. Within days, the customers experienced a marked difference in search results and began finding products faster. Search efficiency soared by 60%, with users finding the right products with greater ease. By manufacturer’s own account, user satisfaction with the search experience jumped a staggering 80%!
The success story though is more than just numbers – it’s a testament to the transformative power of AI in revolutionizing customer experience and driving business growth. To my mind, it highlights the importance of combining different AI techniques like NLP, GenAI, and lexical and semantic search. We also learned the importance of using diverse data sources while ensuring data quality and consistency. Finally, the project underscores the need for continuous testing and refinement to guarantee GenAI’s reliability and effectiveness.
This story is a text-book example of how Generative AI can transform the way people interact with products online. We believe this is just the beginning. As generative AI continues to evolve, we can expect even more exciting possibilities for it to solve numerous other business challenges in the realm of digital enterprise.