Deploying Generative AI: Overcoming Challenges
Overcoming Data Bias, Cost, Use Case, and Adoption Challenges
Strategies for Successful Generative AI Deployment
Generative AI systems, which can autonomously generate content like text, images, music, and videos, are making significant strides in the world of artificial intelligence. As their capabilities continue to evolve, so do the challenges associated with deploying them in the enterprise. In this article, we will explore some of the most pressing challenges that organizations currently face when deploying GenAI systems and discuss strategies for overcoming them.
The biggest challenges in deploying GenAI, as we see them today, are: Data Privacy & Security; Addressing Hallucinations; Computational Costs; Understanding the Right Fit for Use Cases; and Driving Adoption. Let’s delve into each challenge along with possible strategies for addressing them.
Understanding Data Bias in Generative AI Models
Addressing Cost Concerns in Generative AI Projects
Selecting the Right Use Cases for Generative AI
Overcoming Adoption Challenges in Generative AI Implementation
Mitigating Data Bias in Generative AI Training
Optimizing Cost Efficiency in Generative AI Projects
Identifying Use Cases with High ROI for Generative AI
Driving Adoption and Engagement with Generative AI Solutions
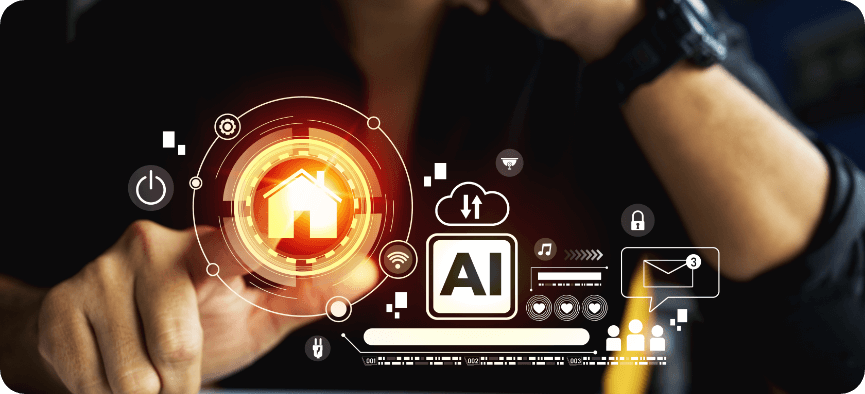
Data Privacy & Security
Data Privacy & Security is perhaps the biggest challenge facing enterprise deployments today. Generative AI systems can be used “as is” for some applications without requiring training. However, for enterprises that want to train Generative AI on their own private data, which often contains sensitive and personal information, there are significant data privacy and security concerns. These concerns include data breaches, unauthorized access, and misuse of the generated content. To address this issue, enterprises can explore a combination of the following:
Addressing Hallucinations
Hallucinations are a significant concern in generative AI systems, especially in the context of text generation. These systems can sometimes produce content that is biased, offensive, or outright false. This can have serious consequences, including spreading misinformation and harming reputations. To address hallucinations, consider these strategies:
Computational Costs
The computational demands of generative AI systems are substantial. Training such models requires powerful hardware and can be an expensive proposition. Besides, there can be additional challenges in deploy these models in resource-constrained environments. However, computational costs can be overcome via:
Understanding the Right Fit for Use Cases
When it comes to deploying generative AI systems understanding where and how they can be effectively applied can be surprising difficult. For not all use cases benefit from such systems, and in some cases, traditional rule-based or manual approaches often make better sense. Hence to determine the right fit for use cases, enterprises can:
Driving Adoption
Generative AI systems in the enterprise are still a relatively new phenomenon and lack of precedents can often make user adoption challenging. User resistance to change, lack of expertise, and concerns about job displacement are just some of the concerns which can hinder its wider adoption. Overcoming user resistance, however, is possible through:
In conclusion, while generative AI promises immense potential, successfully deploying these systems in the enterprise involves navigating a variety of challenges from data privacy to driving adoption. However, by taking a proactive approach, leveraging strategies like robust data protections, human-in-the-loop reviews, change management, and pilot projects, organizations can overcome these hurdles. With careful planning and execution, enterprises can unlock the tremendous benefits of generative AI to drive innovation and efficiency across diverse use cases.