Unveiling the Future of Fintech: The Dominance of Analytics
Leveraging Data Analytics: Driving Innovation in Fintech
Charting the Course: Navigating the Future Landscape of Fintech Analytics
Fintech is slowly taking over the world, with 46% of consumers using digital banking channels exclusively. In 2020, the global fintech market was valued at $110.57 billion — a figure that is expected to grow to $698.48 billion by 2030.
Of all the things contributing to this bright future for fintech, data analytics is one of the most important. It’s what allows fintech companies to make quick, accurate decisions in order to better serve their customers.
Understanding the Role of Analytics in Fintech Transformation
Exploring Emerging Trends and Opportunities in Fintech Analytics
Overcoming Challenges: Harnessing the Power of Data Analytics in Fintech
Case Studies: Success Stories of Analytics Integration in Fintech Solutions
The Evolution of Fintech: From Traditional Banking to Data-Driven Innovation
Opportunities for Growth: Key Areas Where Analytics Can Revolutionize Fintech
Addressing the Challenges: Strategies for Effective Implementation of Analytics in Fintech
Future Outlook: Predictions and Projections for the Role of Analytics in Fintech
In 2022, the global big data industry is valued at $274.3 billion, and this valuation is expected to increase exponentially in the future due to the generation of nearly 2.5 quintillion bytes of data by global internet users every single day.
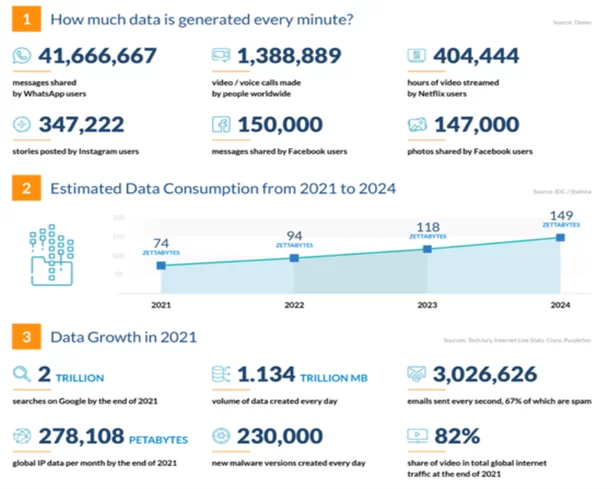
The concept of Big Data is based on principles that might be called the three Vs:
- Volume: Traditional technologies cannot collect such a tremendous amount of data, so there must be special methods and technologies for Big Data collection.
- Velocity: Data needs to be processed in real-time to get up-to-date information.
- Variety: Technologies should be able to process a great variety of data formats, such as audio, video, and even tweets.
Data gives organizations the power to make informed decisions and boost revenue generation. By efficiently tracking, collecting, and analyzing actionable, data-driven insights, companies are able to remain competitive, deliver better products and services, improve customer satisfaction, and drive innovation. Analytics allow key players in the fintech industry to:
- Make Informed Decisions: Fintech companies can use data to understand consumer behavior and act on it accordingly. In this way, fintech corporations can make better-informed decisions about what products and services to offer and how to market them to customers.
- Generate Revenue: Fintech companies can use big data to identify new opportunities for revenue generation. They can also track customer spending patterns and understand what products or services are most appealing to them. The information can then be used to increase sales and boost profits.
- Improve Operational Efficiency: The inherent mechanism of most workplace operations can be improved by taking appropriate action on the data collected from internal and external sources. Fintech companies can use data analytics to identify areas of inefficiency and augment their operations accordingly.
- Improve Marketing: Analytics can help businesses understand which marketing efforts are working and which ones need to be changed or dropped altogether. Fintech companies can use data to identify customer segments, understand their needs, and craft targeted marketing campaigns across multiple channels.
- Identify Trends/Frauds: Trend detection depends largely on data analytics, and fintech companies can leverage it to make sure they’re one step ahead of the competition. Likewise, fintech lending can benefit from analytics’ fraud and risk detection capabilities.
- Reduce Business Threats and Financial Waste: Financial waste occurs when companies make uninformed decisions. Companies that lack information often lag behind their competitors. Meanwhile, data-backed decisions can help mitigate business threats and reduce resource waste in the fintech world.
With every passing day, our world’s reliance on data is increasing. Businesses in the fintech industry need to know how to collect, track, organize, scale, and use analytics to ensure success and growth.
Importance of Big Data in FinTech Industry
Big data is in high demand in finance for a variety of reasons:
A. Lack of Personal Connection with the customers.
Users expect to be able to address their problems without having to go to a bank office, but this makes gathering information on clients more difficult. Mobile gadgets can be of assistance.
They let companies gather many sorts of information, such as geolocation, the most common user interactions, user behavior, and browsing history. This information may then be utilized to make up for a lack of face-to-face connection with clients.
B. FinTech’s social media footprint is growing.
Users make purchases and engage with companies using social media, which is no longer only a platform for connecting with friends and family. Examining user behavior on social media is critical for FinTech firms to gain insights and apply them when selling products or services. Insurers, for example, may create unique plans based on social media data, and banks can use social media data to create credit scores.
C. Expectations of customers are shifting.
Customers want businesses to not just meet their requirements, but also to anticipate and surpass them. This is impossible without consumer information. To provide tailored offers for clients, a FinTech company should collect data from numerous channels such as their mobile app, website, wearables, social media, and smart devices.
The client experience has altered because of online banking. You no longer need to visit physical sites or wait days for transactions to be completed. Fintech’s have developed cross-border financial services that enable real-time data sharing. It allows buyers and sellers to conduct business without friction.
Fintech has driven conventional players to adapt by allowing for more personalized alternatives in financial services. Fintech firms may be more nimble and respond rapidly to changing market conditions since they are founded on contemporary technological platforms.
D. FinTech is becoming increasingly competitive.
The FinTech sector is quickly expanding, attracting an increasing number of entrepreneurs, startups, and established businesses every day. The ability of a FinTech product to deliver a service is critical to its success in this competitive industry.
Big data enables businesses to optimize their operations in real-time and provide their customers with the finest services possible based on hard facts.
Reduced operating expenses allow firms to dedicate resources to marketing and decrease pricing for customers, allowing them to stay ahead of the competition. As a result, FinTech companies must automate their operations in order to save money, and big data insights can assist.
How Fintech Companies Are Using Data to Better Serve Customers
Algorithms, Artificial Intelligence (AI), and Machine Learning (ML) can process the vast amount of data that is generated every day and create actionable items. The data that is shared can be used to predict and anticipate customer behavior. This allows companies to share potential products, upsells, cross-sells, and strategic planning to customers.
A. Segmentation
The more fintech institutions know about their customers, the better than can serve them. In addition to checking credit scores and other hard credit variables, segmenting your potential customers can help you target marketing and services.
Segmentation sorts of customers by various categories and can provide data about what products meet the needs of its customers.
- Demographics (Age, Gender, Education, Location)
- Family (Size, Dependents)
- Employment (Years in business
- Financial (Income, Net Worth, Assets)
- Online behavior
Segmentation can also help identify the high-value customers that are most likely to purchase financial products.
It also helps to understand customer needs and provide more personalized offers.
B. Understanding Customer Needs
76% of customers now expect companies to understand their needs and expectations. Big data allows you to know customers now better than ever. The amount of data generated by credit card transactions, ATM withdrawals, credit scores, and other financial tools is staggering. Using this trove of information, fintech businesses have a deeper insight into their customer’s needs.
This knowledge also helps to personalize offers directly to targeted customers. Customers pay more attention to personalized offers.
When it comes to doing business with someone, trust is crucial. People are judging a company’s credibility and trustworthiness by the quality of their user experience. Increasingly, that experience is mobile. If your app doesn’t perform as advertised or is not intuitive to use, it will negatively impact their experience and their trust. Big Data lets companies continuously optimize their customer’s online experience.
C. Managing Risk
A big part of success in the fintech industry is about managing risk. Data analysis can help identify potential bad investments or flag customers that are showing warning signs of trouble.
D. Detecting Fraud
Part of managing the risk is quickly identifying fraud when it occurs. Credit and debit card fraud alone accounted for $14.7 billion in losses for the financial industry in 2018. Even a small reduction in losses can add up quickly.
AI and ML are now flagging potential fraud even before it occurs. By analyzing the spending habits of customers, it can identify purchases or locations that do not fit the profile and flag them for review before completing transactions.
E. Compliance
Tech can also help when it comes to compliance. In addition to tracking transactions, AI can analyze and detect where financial irregularities are more likely to occur. This allows fintech companies to take preventative measures before a problem grows.
F. Chatbots, Bots, and Robotic Process Automation
AI-enhanced Chatbots provide 24/7 interactivity. These smart virtual assistants can handle transactions, provide important information, and help customers in a variety of ways.
Robotic Process Automation (RPA) is improving the user experience by allowing bots to handle repetitive (and labor-intensive) tasks without human intervention. Not only does it reduce errors, but it frees up team members to handle more complex queries and provide better customer service.
How big data helps fintech companies and startups to better serve and protect their customers
There are several distinct areas where financial technologies and big data form a perfect symbiosis that leads to significantly better outcomes for service providers and consumers alike.
A. Big data processing
- Real-time data processing facilitates the task of tracking users’ activities in web and mobile applications for subsequent analysis.
- Data mining helps take a deeper dive into large volumes of unstructured data stored in data lakes, drawing actionable insights from them.
- Data visualization on customizable dashboards provides a way to get a clear view of the most important aspects of key business processes.
- Stream computing systems merge data streams from applications and Internet of Things networks, analyzing them on the go and returning clean, usable data sets.
B. Data analytics
- Advanced risk analysis algorithms rely on artificial intelligence and machine learning methods to achieve better accuracy.
- Data-driven decision making is based on big data analysis and AI/ML algorithms that suggest the optimal course of action in complex situations.
- Business process automation coupled with intelligent decision-making reduces service delays and improves the customer experience.
- Continuous social analytics help monitor the brand image in social networks and detect issues at an early stage, thus enabling marketing teams to maintain a consistently high CSAT (customer satisfaction) score.
C. Data security
- Big data helps build very detailed customer profiles that are used for reliable protection of users’ identity and personal data, which is especially important for financial institutions.
- AI and big data are widely used for detecting suspicious activities and preventing various types of fraud and elaborate hacking attempts.
In principle, these and other related practices and approaches form the data management framework of any modern digital bank or financial technology company. Big data collection and 360-degree data analysis are no longer viewed as a fad that reflects the latest fashion in computer science. On the contrary, they are essential components of any institution working with money and striving to be effective in a very competitive landscape.
Big data use cases in fintech
Big data in fintech companies is truly ubiquitous and actively used in different contexts for a broad range of purposes. Let’s take a look at a few examples of where combining the use of big data and associated technologies makes the most practical sense.
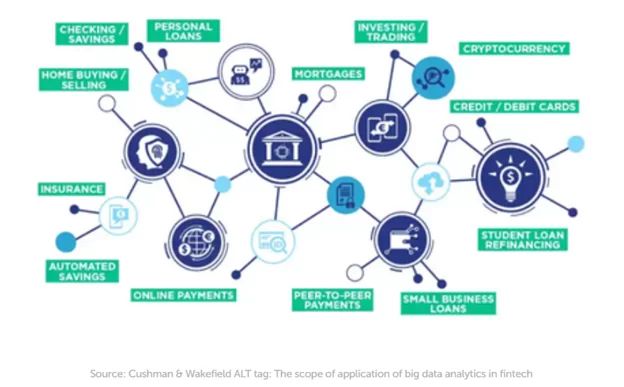
A. Fraud Prevention
Fraudsters stealing company data and money is a nightmare scenario for every financial organization. So, they’re using every anti-fraud tool available to defend information.
For example, companies can implement Regulatory Technology, or Regtech, to strengthen security systems. This includes:
- intelligence applications for Anti-Money Laundering (AML).
- a single-risk platform for end-to-end fraud protection.
- blockchain technologies for increasing the safety of direct transactions.
- Machine Learning for communication monitoring and customer identification.
Also, Machine Learning algorithms can analyze the latest consumer experience and compare it to all previous activities. This enables organizations to proactively react to unusual actions.
By the way, small banks and credit unions are more likely to be attacked by fraudsters than bigger financial institutions. So, the combination of financial data analysis and Machine Learning analytics is an important means of protecting financial organizations of all sizes and their financial databases from falling victim to fraudulent activities such as speculator trading, rogue trading, and regulatory violations.
B. Risk Analysis
Data Science provides an organization with deep risk analysis and helps manage risks by implementing new features of risk detection systems.
Organizations can prepare for potential problems by simulating particular risks in a virtual environment. For example, they can perform risk analysis to see whether a potential borrower is likely to actually repay the loan. Risk simulation helps companies to understand the risk preconditions and monitor them effectively across the corporation.
Data Science creates a risk model based on the history of consumers’ transactions. It helps to determine if users are reliable and, if so, can offer them benefits such as lower rates and additional services.
Also, risk assessment via Data Science can improve audit management, as creating a risk assessment plan is a vital part of the auditing process.
C. Customer Behavior Analysis
Data Science analyzes a large amount of behavioral data using text analysis, natural language processing, and data mining.
Customer behavior analysis allows financial companies to:
- boost cross-sales by offering customers additional products or services of interest.
- estimate and increase clients’ lifetime value.
- reduce losses by decreasing below-zero-value customers.
- divide customers into segments.
- enhance the business’s reputation as a trusted partner.
Data Science combines the opportunity of creating customer behavior models and conducting real-time and predictive fintech analytics.
D. Credit Allocation
In this case, fintech Data Science analyzes banking databases and open financial data provided by clients along with their credit history. Also, particular banks have the right to exchange some financial information, which provides the opportunity to gather more data about clients.
This helps banks automate the process of defining the credit score of each client. No manual labor is required to predict whether the client is a good candidate for a loan.
Moreover, Machine Learning can match users’ behavior to credit scoring models and predict how likely the customer is to repay the loan.
E. Predictive Analytics
Predictive analytics extrapolate from current data to forecast future trends. Thus, fintech Data Science can help to predict stock trends and market prices and help users make wise investments or develop financial strategies.
F. Product Improvement
One more way of using Data Science is to create product improvement strategies. It helps organizations adapt to changing market demands, understand the weaknesses of the company’s products, and modernize them.
Data Science, combined with Artificial Intelligence, allows financial firms to introduce new products to users at the most favorable time when the market is the most responsive.
G. Algorithmic Trading
Algorithmic trading is a set of instructions programmed to solve trading issues, taking into account variables such as price, timing, and volume.
In developed markets, today around 70-80% of all trades are algorithmic, and these numbers are expected to grow up to 95% in the next few years.
Algorithmic trading uses mathematical formulas and models, along with human oversight, to find even the least obvious opportunities for successful trading. This combination allows firms to define whether it is effective to trade on a particular market at a particular time.
The big benefit of using special fintech Data Science applications is the speed of actions and reaction to the consequences. The algorithms ensure that if a particular step turns out to be wrong, the program will correct the decision-making model, taking the mistake into account.
H. Personalized Marketing
Thanks to in-depth analysis of trends and customers’ preferences, financial companies can offer personalized ads and products for each segment of users.
Personalized marketing campaigns help to reach the target audience, improve customer retention, and make for a better user experience.
Personalization also improves the conversion rate and increases the return on investment, leading to better financial performance of the organization.
As you can see, financial data analysis can be used in a broad variety of spheres in the fintech industry. But a lot of attention is given to the correlation between Big Data and customer experience. We’ll explain that correlation below.