Value Your Data: Understanding Data as an Asset in Modern Business
Leveraging Data Assets: Best Practices for Effective Data Management
Maximizing Business Value: Strategies for Data Asset Optimization
With the advancement in the capturing, storage, and processing technology; our world is generating 2.5 quintillion** bytes of data every day. 90 % of data being produced in last 2 years. And digital platforms are leveraging this data to reap the benefits.
Let us quickly shift focus to “How valuable the data can be!”
For this let us look at one of the hottest startups in India’s credit card payment market, CRED. CRED in nutshell is the platform (Data Driven!) with unique business model and simple yet important function to help customer to manage their credit card bills avoiding their late fees and interests.
CRED platform has partnered with various business (generally lifestyle) which provides the offers to CRED users. And user can burn their CRED coins which they receive in turn while they make their credit card payment through the CRED app. Revenue earned AS OF NOW is through businesses who pay fees to post the offers. Future potential of CRED is becoming a Bank serving spending population of India. Valuation of CRED stands at 2.2 billion USD. “This is how valuable your data can be!”
The Importance of Data Management: Unlocking the Value of Your Data Assets
Building a Data-Driven Culture: Empowering Organizations with Valuable Insights
Implementing Effective Data Governance: Ensuring the Integrity and Value of Your Data
Driving Business Success with Data: Key Principles and Strategies for Data Asset Management
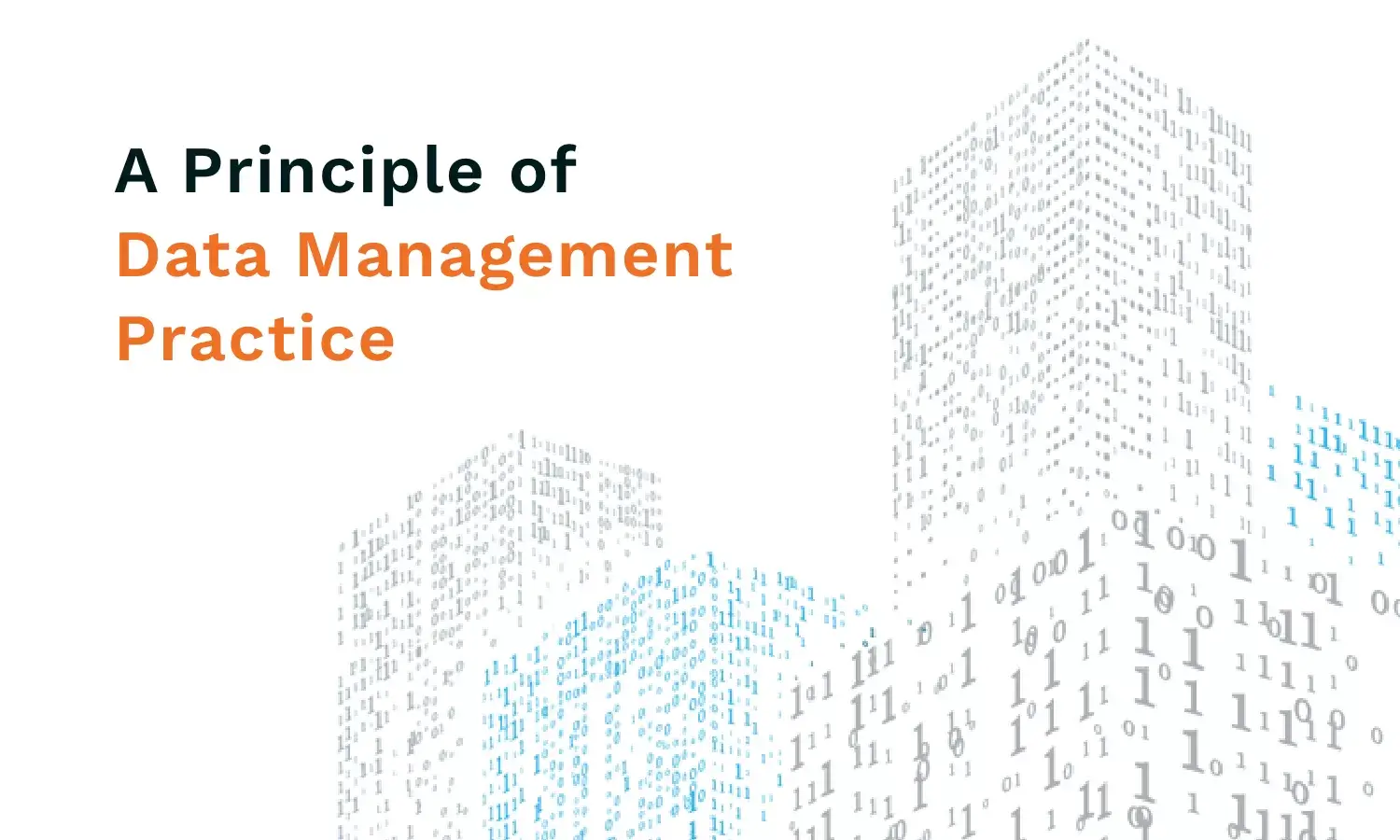
The same mindset of data valuation should flow in inward in an organization to have data driven organization. Decision when made along with data is always more effective and efficient not to run the business but also to strategize and innovate. Data can give you unveil the hidden truths in gaps in operations. It can help an organization to strategize new services/products. It can also help companies to compete with others through innovation.
Treating data as an asset is not only responsibility of CIO/CDO, but business is also equally responsible to make an organization data driven (CIO/CDO becoming the primary driver).
Data as a Strategic Asset: Transforming Information into Business Value
Enhancing Data Quality: Ensuring Accuracy, Consistency, and Relevance
Data Governance Best Practices: Establishing Policies and Processes for Data Asset Management
Measuring Data Value: Metrics and KPIs for Evaluating the Impact of Data Assets
Data is very different beast to manage as compared to Money/Commodity. Money and commodity can be touched, can be consumed/utilized, can be enjoyed. So, it becomes very easy to value a money or commodity while data is little different to value.
Data possesses unique drivers though for valuation.
- Exclusive The unique the data more valuable. The use case defines the value of the data.
- Risk The potential risk associated with the data will reduce the value of the data.
- Accessible The accessibility of data is very important; the more data is accessible more the usage.
- Governed/Restricted Less restricted is the data higher the value. This also defers for each use case.
- Timed% The data received on time has more valuable. The value in timeliness depends on use case.
- Accurate Accuracy of data stands very important to rely on data. More reliable the data more valuable it is.
- Consistent Data is said to be consistent if it follows certain defined patten and format.
- Complete More the complete data more valuable it is.
Apart from drivers specified above, data also poses few economic characteristics as mentioned below.
- Data is non-rival: Data can be used by multiple parties without it being used up or depleted.
- Data has exclusiveness embedded: Data can be for public good, personal good, or enterprise/org good based on the information it contains. Same data can be shared with everyone while other is exclusive to certain people or groups.
- Data involves externalities: Externalities means profit or loss incurred based on certain decisions. Data can create externalities certain data can leads to insight to mitigate risk and/or profit in some case data leak can lead to havoc.
- Data may have increasing or decreasing returns: Collection of more data can lead to better insights or sometimes it can just lead to extra cost.
- Data has large option value: Data also have future value. Organization can choose to collect/store/use data based not only on current value but also future value. The value of data is highly fluctuating in certain cases.
- Data collection generally have high Capex and marginal Opex: Initial investment for data collection/storage/usage generally requires upfront investment and post that it marginally incremental cost which is generally very less.
- Data use requires complementary investments: Organizations may need to invest in software, hardware, and personnel in order to realize value from data. If organizations can assess the valuation of data against this driver in context of source applications and use cases, they will be able to realize the potential of data as economic benefits.
How to Value your Data
Valuing data is a multi-stepped process. Each step required thorough understanding of data (generated/stored) in an Enterprise into consideration.
The steps are.
- Identify and create an inventory/catalog of the current data assets.
- Identify data attributes attached to data assets.
- Identify current use cases.
- Valuation
- Evaluate the current use cases assessing the drivers of the data assets.
- Evaluate use cases with possible future valuation.
- Document the future use cases for further use.
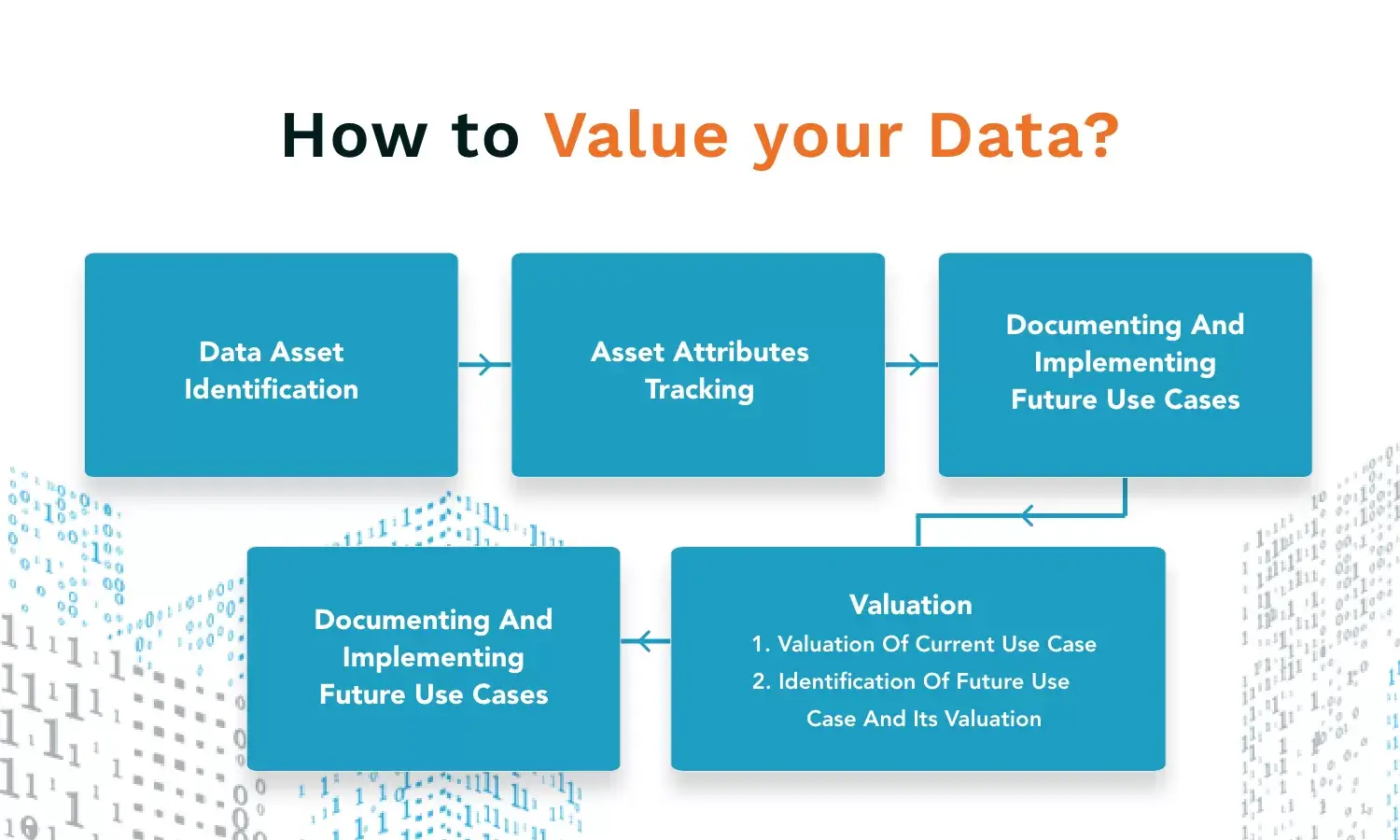
Methodologies of Data Valuation
Due to constantly changing nature of the data, there is no standard methods of data valuation. Though there are few models that can be leveraged to estimate the value of data. The most widely used are developed by Doug Laney. Doug Laney has divided the methods based on nature of benefits namely Foundational Models and Financial Models.
We can summarize this model as below.
Foundational Models
- Intrinsic Value of Information (IVI) – This model is aimed to measure the drivers like correctness, completeness, and exclusiveness of the data.
- Business Value of Information (BVI) – This model is aimed to measure the fitness of the data for specific cases considering accuracy, frequency, and completeness.
- Performance Value of Information (PVI) – This model is aimed to measure on data value to drive key business drivers. Generally, use case in controlled group.
Financial Models
- Cost value of Information (CVI) – This model is aimed to measure cost too produce and store data, the cost to replace the data or impact on cash flow if the data is lost.
- Market Value of Information (MVI) – This model is aimed to measure actual or estimated value to data if traded into marketplace.
- Economic value to Information (EVI) – This model is aimed to measures the expected cash flows, returns or savings from the usage of the data.
Few other valuation methods that are widely used for valuation of data are mentioned below.
Modified cost value approach
This approach is based on cost of recreating or replicating the data. It suggests some modification.
- Redundant data has zero value to avoid double counting.
- Unused data has zero value.
The value of data should be depreciated based on the calculated “Shelf Life”.
Consumption based approach
This valuation is built on principles of Modified cost-based approach. The weightage is applied based on the data asset contributes relative value to the organization.
Data Hub valuation
This valuation approach is same as the cost-based approach only difference being scope is not at each data point level rather the valuation is done for group of datasets called data hubs. Another method is to check the value the external market is ready to pay for the data hubs.
Stakeholder approach
This approach involves key stakeholder to value the data, by identifying how data supports activities which external stakeholders identify as creating value for them. It uses a model that combines the total value created by the organization, a weighted list of value creating initiatives (as defined by external stakeholders) and an inventory of data assets.
In a nutshell, data is extremely valuable, and an organization need to build an ecosystem inclusive of Business and Technology teams to mine the right value out of it.