Key Trends and Focus Areas in Artificial Intelligence (AI) and Machine Learning (ML)
Exploring the Future of AI and ML: Trends and Developments
The Evolution of Technology: Insights into AI and ML Trends
I have been in the Data Field for more than a decade and from my understanding, organizations that are strengthening their AI/ML capabilities will focus on the following key areas.
Understanding the Landscape: Key Trends in AI and ML
Emerging Applications: How AI and ML Are Reshaping Industries
Driving Innovation: Exploring the Potential of AI and ML
Navigating the Future: Strategies for Leveraging AI and ML in Business
Post-Pandemic Reset
Most of the predictive models that are currently in production and making predictions have to be reset. The historical data used to build these predictive models may no longer be valid in the post-pandemic market. It doesn’t mean that the current models are obsolete. But they need to be retrained (parameter tuning) to accommodate the pandemic conditions. Alibaba’s Online platform already tweaked its ML models and was able to achieve significant sales during the 2020 Single’s day sale. Every organization will be looking for such resets to their Predictive algorithms.
Machine Learning Operations (MLOps)
When more and more ML algorithms are deployed across the organization and operationalized, the measurement of model performance of the model is very important. Every ML model will go through Data Drift, Mathematical Drift, or Business Drift. This way the model’s performance will dip and we need to identify the same as early as possible. Otherwise, the results from the model will be skewed and will become obsolete. Using an appropriate MLOps platform to build and operationalize an effective ML pipeline and smooth operations is very important.
Information Architecture (IA)
Building Advanced Analytics and creating advanced AI systems is not possible without a robust “Information Architecture”. IA will set the base for enterprise-level AI. This involves connecting various data silos across the organization landscape through various techniques and best practices. Once this is established, then the analytics journey starts at an enterprise scale.
Decision Intelligence (DI)
While more and more Algorithms are deployed into production, organizations will start investing in decision intelligence capabilities. This brings together several disciplines across the organization including decision management and decision support. DI team will work with various other internal teams and will become responsible for supporting every decision taken by AI models.
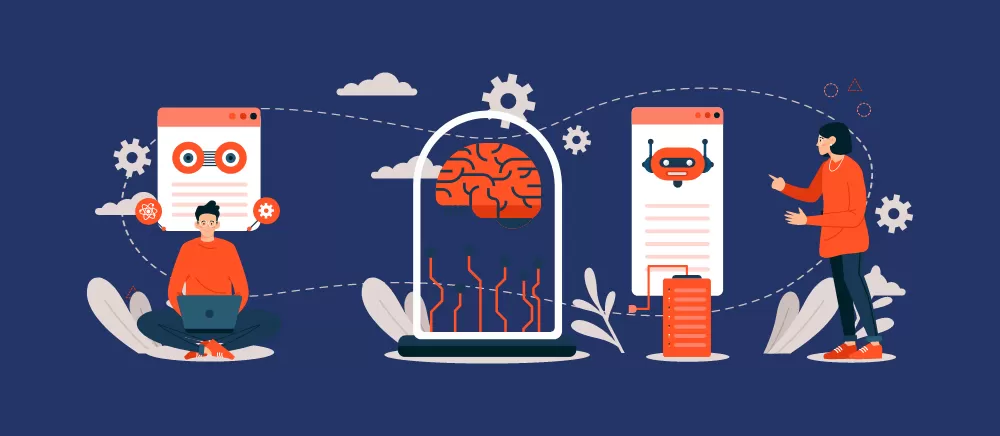
Ethical AI
An organization’s ethics is defined by how the organization responds to an internal as well as external stimulus. Ethics are the values of an individual to help govern his or her actions and decisions. Every individual’s work decision is closely tied to organizational Ethics. Organizational values are defined based on these. On the other hand, AI refers to the simulation of human intelligence in machines that are programmed to think and act like human beings. How do organizations take accountability for AI’s decisions? We have all heard about several cases of failures such as AI-based Chatbots malfunctioning and behaving in a biased manner. These systems when they make mistakes, Organizations have to take responsibility for their failures.
Organizations will work on the ‘Ethical AI framework’ to prove their AI models are free from various ‘Bias’ issues and act with all ‘Fairness’.
Similarly, Data Scientists and Practitioners will be focusing on the following areas:
New Learning
Today most of the algorithms in analytics solutions are based on Supervised learning. But it is also difficult and time-consuming due to ‘data labeling’ activity. There are several teams in large AI organizations that perform only labeling activity. Also, if we see that the world has a huge amount of unlabelled data. Professor “Yann LeCun,” says that in the coming years there will be a new learning method called “Self-Supervised Learning” in which we need to train some portion of the data and set it as a “supervisory signal” for the entire dataset which is unlabelled. This context will help in identifying the patterns in the entire dataset.
Similarly, today’s data is omnipresent, but algorithms need them in one place for training. In many scenarios these data are sensitive. e.g., If a health care organization wants to build a predictive algorithm based on various health vitals data of individuals, they need to collect them in one place. We do have data about an individual’s health, workout, and other data in digital devices like mobile phones, fitness trackers, smartwatches, etc. But due to sensitivity and PII restrictions, organizations will not be able to get these data. “Federated Learning” is a new method in which the algorithm will be shipped to individual devices and gets trained there. Then the trained algorithm just takes the hyperparameters and gets aggregated centrally. The core model in the central system will be retrained with these hyperparameters and tuned.
Explainability of AI
One of the most difficult problems in choosing the final model for production is the ability to interpret the model. While we all know that “black box” models (e.g., Random Forest, Neural Nets) are more accurate (whatever parameter we want to use for evaluating the model performance and stability) than the “white box” models (e.g., Linear Regression, Decision Trees), in many situations, we end up using white-box models in production. This is because it is easy to explain the output based on the input in white-box models. New methodologies such as LIME, SHAP are evolving which will help in understanding the model and hyperparameters.
AI Integration
As 5G and EDGE analytics are right around the corner, one of the key trends will be how to integrate AI models into the EDGE devices and other smart devices. More integration work in importing models into these devices will happen. They will form a foundational building block for Machine-to-Machine Learning (M2ML) and evolution towards AGI (Artificial General Intelligence) and then to ASI (Artificial Super Intelligence).
Let us take the example of Autonomous cars. In the future, when all the cars on the road are autonomous, the road infrastructure will be very different, in such a way that there may not be traffic signals, speed bumps, etc. Every vehicle on the road must communicate with every other vehicle nearby and they must make decisions in real-time for uninterrupted traffic flow. EDGE analytics will be a starting point for this change.
Industry-Specific Trends: AI and ML Applications Across Various Sectors
Ethical Considerations: Addressing Challenges and Opportunities in AI and ML
The Role of AI and ML in Digital Transformation: Insights and Best Practices
Looking Ahead: Predictions for the Future of AI and ML
How USEReady Can Help
USEReady is an award winning, data transformation consultancy that applies best practices and technology to deliver solutions that help our customers succeed with data. We bring deep expertise and skills in design and engineering of modern Data Science and Machine Learning (DSML) solutions.
Through our holistic approach, expertise in modern data platforms and our Analytics Maturity Framework; we enable customers to navigate through the intricacies of ML journey and accelerate their adoption and deployment.