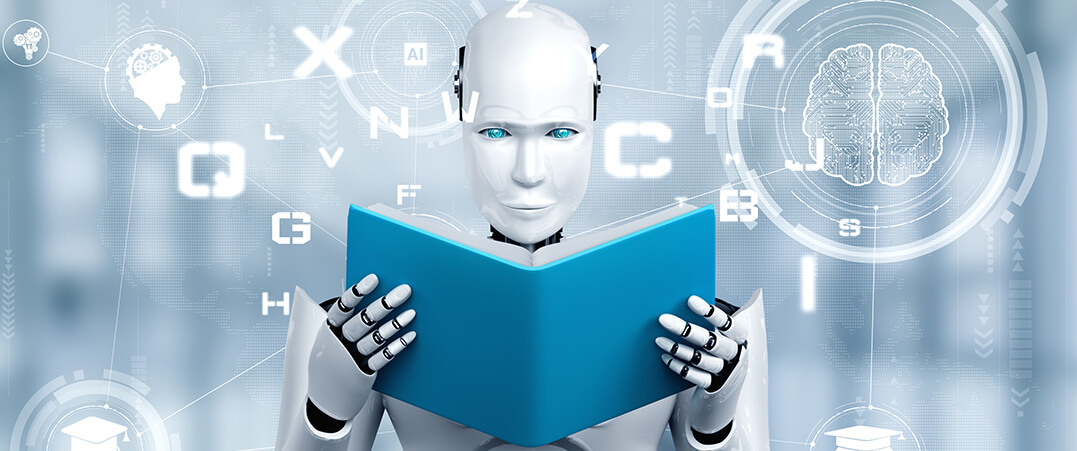
Unlocking Predictive Analytics in Qlik Sense with AutoML
Enhancing Data Analytics with AutoML Integration
Automating Decision-Making in Qlik Sense with AutoML
The Benefits of AutoML in Qlik Sense
Steps to Integrate AutoML into Qlik Sense
Use Cases for AutoML in Advanced Analytics
Improving Decision-Making with Predictive Analytics
Setting Up AutoML for Qlik Sense
Analyzing Data Trends with AutoML Integration
Automating Predictive Models in Qlik Sense
Overcoming Challenges in AutoML Integration
Machine Learning (ML) isn’t exactly easy or common knowledge. It takes time and effort to fully grasp it. It requires more accessibility and efficiency especially for those who don’t possess deep expertise. However, for those still keen on it, integrating AutoML can be quite handy. AutoML is a technique involved in automating the application and training multiple ML models democratizes advanced data analysis, enabling those without deep machine learning knowledge to leverage its power.
AutoML streamlines the process of selecting, training, and optimizing models, thus facilitating the use of diverse machine learning applications across various business scenarios.
Users can use Google Cloud AutoML, H2O.ai, Dataiku, DataRobot, JADBio AutoML and Microsoft’s Azure AutoML frameworks.
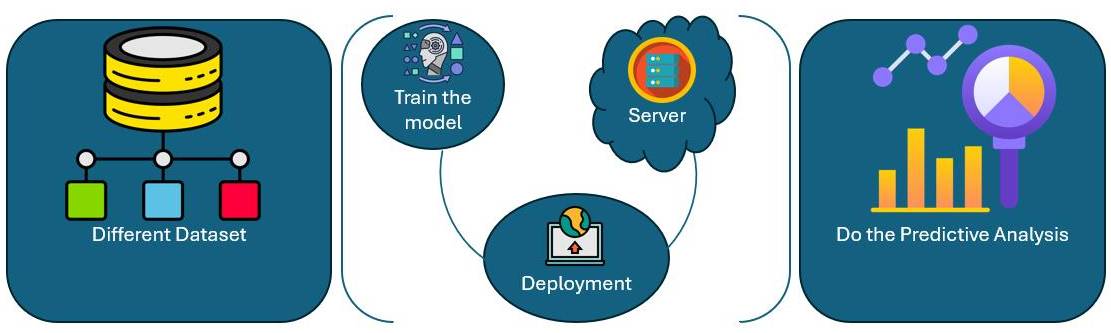
Process Involved in AutoML
Data Preparation
Automates data cleaning and preparation steps, such as managing missing values, normalizing features, and encoding categorical variables.
Feature Designing
Enhances a model’s accuracy and predictive power by automatically selecting, creating, or transforming features to improve performance and robustness.
Model Selection
Automates the identification of the most suitable machine learning algorithms for a specific problem, evaluating various models and configurations to find the best fit.
Tuning of Meta-Parameters
Optimizes the parameters of machine learning models to enhance their performance and control various aspects of the training algorithms.
Model Estimation
Automatically assesses model performance using metrics and validation techniques, ensuring generalization to new and unseen data.
Deployment
Simplifying the process of deploying models into production environments.
Integrating AutoML in Qlik Sense
Integration of AutoML in popular BI tool Qlik Sense can significantly improve its capabilities and its accessibility for users. The need for AutoML in Qlik Sense arises from the growing demand for advanced analytics and predictive capabilities within data visualization platforms.
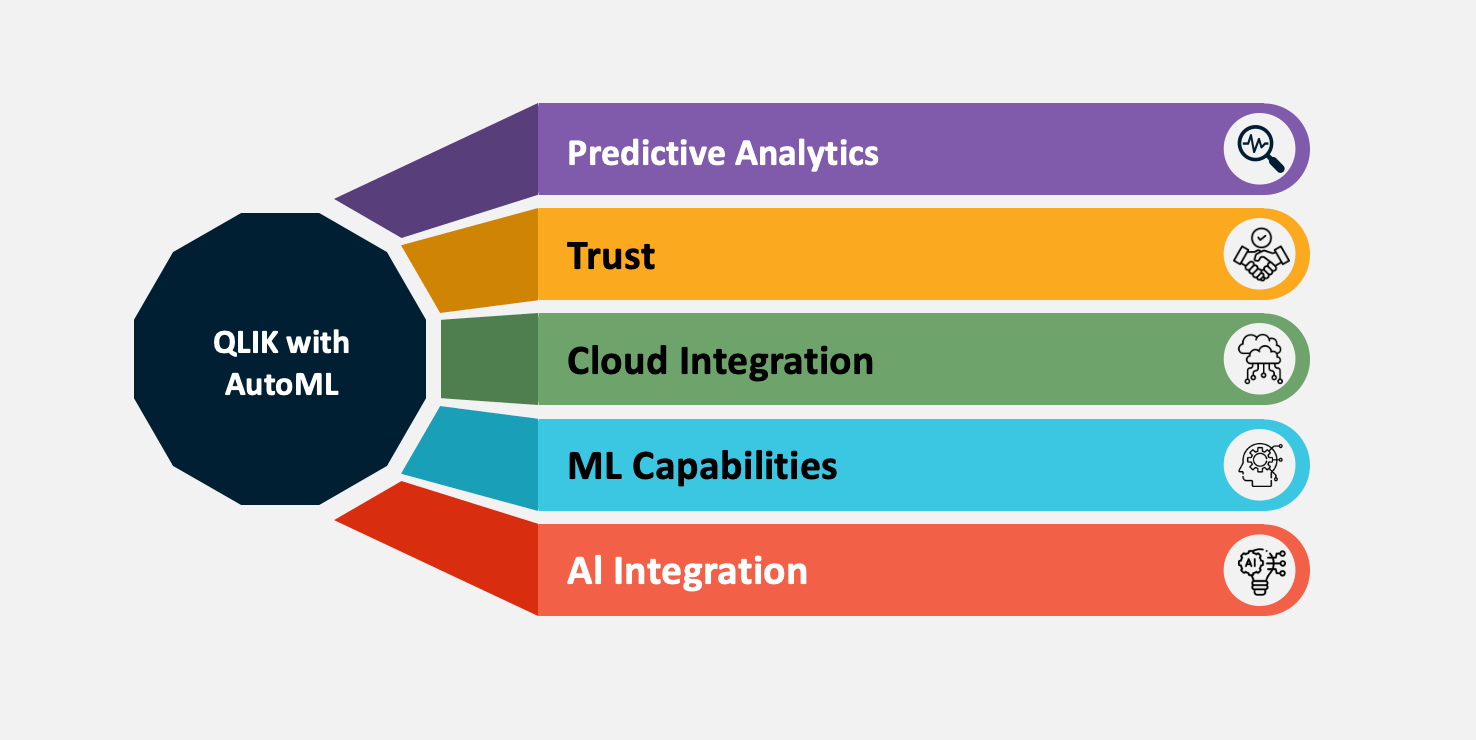
By incorporating these tools into Qlik Sense, users can access advanced analytics features without needing extensive data science expertise.
Benefits of Integrating AutoML with Qlik Sense include:
Enhanced Predictive Analytics Capabilities
AutoML can automate the creation of predictive models, making it easier for users to generate forecasts and predictions without needing deep expertise in machine learning. This integration supports effective business decision-making.
User-Friendliness
Designed for straightforward deployment of machine learning models within production environments.
Increased Capability
AutoML can eliminate the complexities from the tasks like preparing the information, attribute selection, model preference, and parameter tuning and increase the productiveness and capability of applications and models. That results in faster insights and more decisive actions.
Improved Accuracy
AutoML platforms often employ sophisticated techniques to optimize models, which can help to improve accuracy and performance compared to manually developed models.
Seamless Integration
The efficient incorporation of AutoML facilitates easy integration with Qlik Sense, allowing users to deploy models directly within the environment. This streamlines workflows and enhances data analysis processes.
Accessibility
AutoML minimizes the limitation to entry for machine learning, making it accessible for business analysts and other non-technical users who may not have a background in data science.
Automation of Model Monitoring
Many AutoML platforms offer features for monitoring and maintaining model performance over time. This can be beneficial for ensuring that the models remain accurate and relevant as new data becomes available.
How to Integrate AutoML with Qlik Sense?
API Integration
Many AutoML platforms provide APIs that can be used to integrate with Qlik Sense. Users can push data from Qlik Sense to the AutoML platform, train the models, and then pull predictions or model results back into Qlik Sense environment.
Data Connectors
Some AutoML tools offer pre-built connectors or plugins for popular data visualization platforms. Users can check if their AutoML tool supports a connector for Qlik Sense.
Export/Import Data
Users can export data from Qlik Sense, use an AutoML tool to build and evaluate models, and then import the results into Qlik Sense for visualization and further analysis as per their need.
Custom Scripts
Users can use Qlik Sense’s scripting capabilities to interact with AutoML models. For example, User can write scripts to automate the data flow between Qlik Sense and their AutoML platform.
Use Cases for AutoML in Qlik Sense:
Sales Forecasting
By using AutoML users can use their historical data to predict future sales trends and other factors.
Customer Segmentation
Automatically segment customers based on purchasing behaviour or other attributes.
Anomaly Detection
AutoML helps to identify unusual patterns or outliers in data that may indicate potential issues and fraudulent activity.
Churn Prediction
With AutoML users can predict which customers will leave or stop using a service, allowing for targeted retention strategies, and proactively minimizing the impact of potential future losses.
Integrating AutoML into Qlik Sense might involve utilizing third-party AutoML platforms that offer APIs or connectors compatible with Qlik Sense. This integration enables users to build and deploy machine learning models directly within their Qlik Sense dashboards, significantly enhancing their data analysis and visualization capabilities.