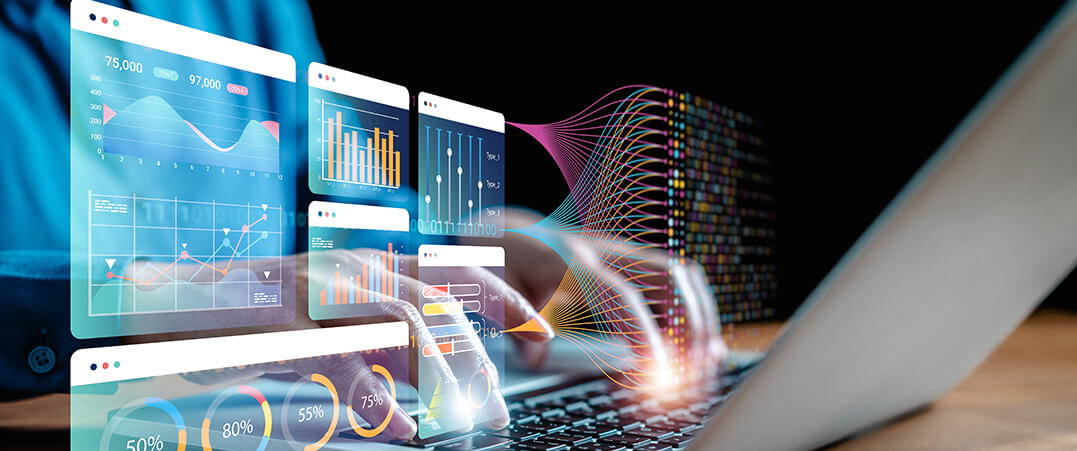
Leveraging ML and Informatica for Data Accuracy
Advanced Data Quality Management Strategies
Machine Learning Solutions for Better Data Quality
The Importance of Data Quality in Modern Businesses
How Machine Learning Transforms Data Cleansing Processes
Using Informatica to Streamline Data Management
Key Benefits of Combining ML and Informatica
Building a Data Quality Framework with Informatica
Machine Learning Models for Identifying Data Anomalies
Ensuring Consistency and Accuracy in Enterprise Data
Overcoming Common Data Quality Challenges
Data quality has long been a challenge for organizations, but its value has only been recognized fairly recently. This shift is largely due to advancements in data warehousing and operational technologies. As data diversity and volume continue to grow, data analysts and engineers increasingly ask themselves, “How can I ensure my data is fit for purpose?” This question has become especially important given the advent of technologies such as machine learning and the vast amounts of structured and unstructured data stored in data lakes.
In today’s data-driven landscape, the importance of high-quality data cannot be overstated.
Gaining a competitive edge in the market, promoting organizational growth, and making well-informed business decisions all depend on having is reliable, accurate, and recent data. Yet many businesses find it difficult to maintain data quality due to its growing volume and complexity.
In this context, Informatica harnesses the power of machine learning to significantly enhance data quality. By employing machine learning algorithms, organizations can detect and rectify data quality issues in real-time, automating processes such as data validation, enrichment, and cleansing. This not only improves the completeness, accuracy, and consistency of data but also enables businesses to make better decisions and operate more efficiently.
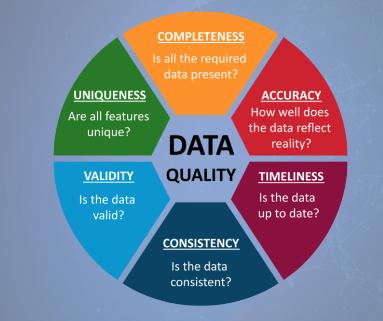
With Informatica, machine learning improves data quality in several important ways, including automatic data cleansing and profiling. Organizations may find and fix data errors before they negatively affect business operations by using machine learning algorithms, which can scan huge volumes of data to find trends, defects, and discrepancies. Informatica ensures that an organization’s data satisfies the highest quality requirements while saving time and money by automating the data cleansing process.
Data Quality and Machine Learning: A Crucial Connection
Informatica leverages machine learning to improve data quality in several critical ways:
1. Data Profiling and Cleansing:
- Content, framework, and discrepancies are found in data by analysing it using ML-powered data quality techniques.
- The performance of ML models can be greatly impacted by incomplete, noisy, and inconsistent data, which they discover.
- Examples of data quality issues addressed:
- Missing Values: ML depends on complete data. Strategies like imputation (mean, median, mode, or k-nearest neighbor) manage missing values.
- Outliers and Default Values: Outliers and default values can change the results; the use case defines which anomalies to include. Accurate data entry is important.
- Duplicates: Finding and eliminating duplicates is necessary to prevent overloading in machine learning models.
2. Automated Data Management:
- Informatica’s platform leverages AI and ML to automate data quality tasks across organizations.
- Features include:
- Automated identification of issues with data quality.
- Proposed data quality rules.
- ML models to enhance procedures for data cleaning.
3. AI-Powered Metadata:
- Informatica enables AI-based data integration and ingestion.
- Artificial intelligence (AI) algorithms suggest the best data sources, connect paths, and transformations to improve output and data quality.
Machine learning is a crucial part of data enrichment in addition to data cleansing and profiling. By utilizing machine learning algorithms to integrate data from other sources, such as social media feeds, market intelligence reports, and geospatial data, Informatica can enhance the quality of data. Thanks to this enrichment process, which enables them to extract crucial insights and context from their data, organizations can make more accurate predictions of future trends and better business decisions.
Machine learning can assist in improving the quality of the data by identifying and resolving issues with data validation. Informatica uses machine learning algorithms to automatically validate data against pre-established business rules, ensuring that it is accurate, consistent, and compliant with regulatory standards. By automating the data validation process, Informatica helps businesses increase the overall trustworthiness of their data while reducing the risk of errors and fraud.
In summary, machine learning is essential for improving the quality of data when utilizing Informatica since it can automate processes like data cleansing, validation, enrichment, and profiling. By enhancing the consistency, accuracy, and completeness of their data, businesses may employ machine learning technology to gain a competitive advantage in the market and improve the quality of their business decisions. The greater the volume and complexity of data, the more important it is to use machine learning in conjunction with Informatica to improve data quality.