Harnessing the Power of Data Virtualization: Simplifying Big Data Projects
Unlocking Big Data Potential: The Role of Data Virtualization in Enterprise Strategy
Streamlining Big Data Initiatives: Leveraging Data Virtualization for Success
In Nov 2017, Gartner predicted that almost 60% of all big data projects fail only to revise it upwards to 85% within a year. Further, Capgemini predicted 70% of big data projects struggle to be profitable/meaningful to the business. One thing is for certain that big data projects are challenging and not for the faint hearted! There are numerous factors at play in this case, rather than just one. For instance, in some cases, it’s the choice of big data storage and processing technologies that makes adoption difficult, while in other cases big data products employed are incorrect to cater to the use case.
In spite the foresaid challenges, organisations continue to launch big data projects in order to take advantage of the immense benefits promised by the big data proponents despite the rather discouraging results. However, the key to success is proper implementation and use of the right tech stack.
Understanding Data Virtualization: A Comprehensive Guide
Benefits of Data Virtualization: How It Enhances Big Data Projects
Challenges of Implementing Data Virtualization in Enterprise Environments
Best Practices for Successful Data Virtualization Deployment
Introduction to Data Virtualization: Definitions, Concepts, and Use Cases
Maximizing the Value of Big Data: Leveraging Data Virtualization Across the Enterprise
Overcoming Common Challenges: Strategies for Effective Data Virtualization Implementation
Case Studies: Real-World Examples of Data Virtualization Success Stories
Key Concepts in Data Virtualisation
Big data project implementations can be made easier by leveraging data virtualisation. However, it won’t solve every issue, but if used correctly, it will unquestionably increase the likelihood of success of big data projects manifold. Below are a few examples.
Big data is stored in plain files in enormous quantities. This makes it challenging for business users with limited technical knowledge to access it. Big data can be made available to a wider business audience and to many well-known BI tools by using data virtualisation services, which can obscure this complexity. They do include big data in easy to access virtual tables.
Data is generated world over by organizations. For instance, at factories, manufacturing plants, or stores; huge volumes of data is created. For downstream applications like reporting and analytics, the quantum of data from different sites makes replication into a single location, simply put unfeasible. To put it another way, big data is harder to move.
Virtual tables that obfuscate the data source can be defined with the help of data virtualisation. To business users, it will appear as though all the data resides in a single repository. Instead of moving large volumes of data to a single location for processing, the data virtualisation server moves the processing to the remote sites.
High-end, transactional NoSQL products are used to store big data in many projects. The majority of these products are built and optimised for handling huge volumes of transactions. Unfortunately, they neglect their analytical and reporting skills in favour of transaction processing. Data virtualisation makes it simple to cache and transfer NoSQL data to any analytical platform. Transactional data may be made quick and easy to access for reporting and analytics thru this process with very little effort.
Big data is not always stored in systems that make it possible to define and document it. As a result, business and technical metadata is frequently absent. A data virtualisation server enables the definition of metadata for many types of data sources in the form of definitions, descriptions, and tags.
The field of big data technologies is evolving at a rapid pace. With focus on governance & security, scale & speed, best practices recommend separation of data consumption from data repositories. A typical case being, in integration of existing data applications with any emerging tech stack becomes difficult if there is downstream/upstream apps incompatibility. In such scenarios, data virtualisation removes the dependencies making it easy to integrate different applications, thereby makes switching to a new, quicker technology a lot easier, quicker and seamless. This helps organisations become less reliant on technologies that could easily become outdated in this fast-paced tech space.
How Data Virtualisation Can Be Useful for Organizations?
In conclusion, organizations will always strive to build big data systems. It’s business critical for them to be data-driven, and for the majority of them, it’s a key step in their digital transformation journey. However, experience has proven that creating big data systems is challenging. Failure to meet business objectives is very real and big. Although data virtualisation servers cannot be the answer to all challenges that come along with digital transformation journey, there are certain crucial ones that they can be addressed to streamline big data projects and make the most of an organisation’s big data investment and help them achieve their business goals. Big data can become simple to use owing to data virtualisation.
Organisations must consider how to manage their big data repositories and provide users access with speed, scale & agility to newer data sources like data lakes, lakehouse or other big data structures. Without it, the repositories run the risk of developing into “big data silos,” which would complicate rather than simplify the information landscape.
Big data fabric becomes useful in this situation. A “platform that accelerates insights by automating ingestion, curation, discovery, preparation, and integration from data silos” is how Forrester describes a big data fabric. Certainly, you must consider how you will build your big data repository, but even more significantly, you must consider how your users will access and use this data in order to acquire those business insights.
Cloud & data consultants from USEReady had implemented a data fabric using virtualization servers to solve business problems similar to the ones discussed here at a global manufacturing giant.
Representative solution implemented shown below.
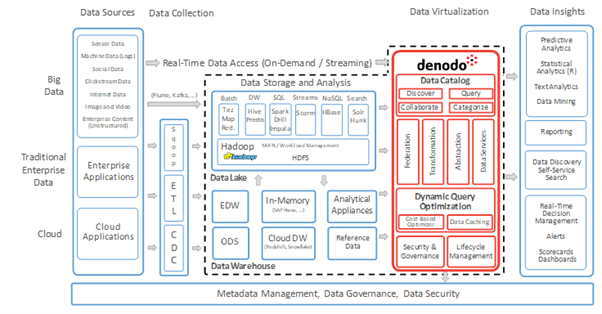
This solution employed Denodo to develop a data virtualization server. Below highlighted are a few key architectural concepts of Denodo.
- A layer for ingesting data referred to as “Data Collection” in the standard architecture.
- A processing and persistence layer referred to as “Data Storage and Analysis” which goes beyond merely being Hadoop-based by incorporating other conventional RDBMS based data processing and storage methods, including a data warehouse.
- A layer for data virtualisation consisting of data access as “data services,” data access through data catalog, data integration and transformation with optimized performance for big data situations, and data security, governance, lineage, etc.
Reference Links:
https://www.capgemini.com/consulting/wp-content/uploads/sites/30/2017/07/big_data_pov_03-02-15.pdf