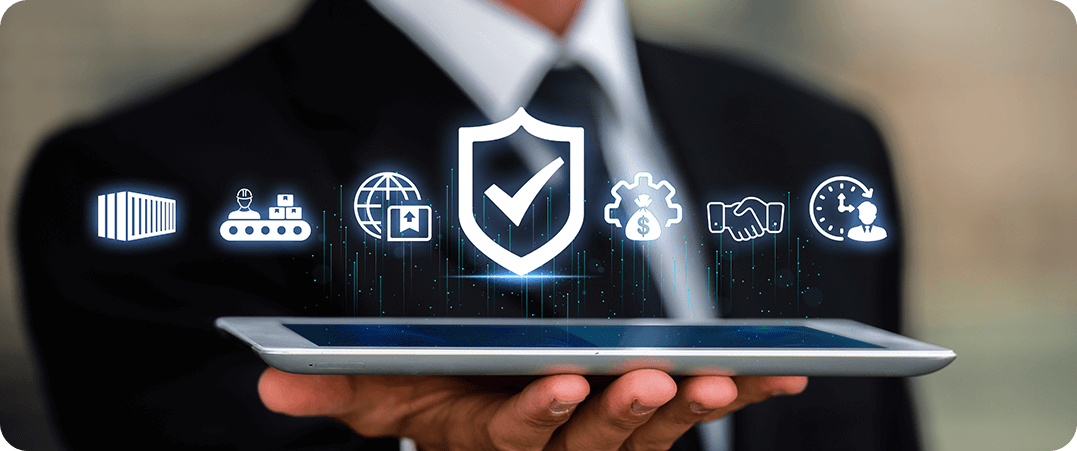
The Importance of Data Governance in Modern AI Strategies
Ensuring AI Success Through Robust Data Governance
Data Governance: Foundation for Reliable AI
Key Principles of Effective Data Governance
How Data Governance Supports AI Initiatives
Data Quality and Integrity for AI Success
Building a Strong Data Governance Framework
Addressing Data Compliance for AI
Enhancing Data Quality for Reliable AI Outcomes
Developing a Governance-Driven AI Strategy
Overcoming Common Data Governance Challenges
In 2024, companies are more committed than ever to enhancing their Data Governance (DG) programs. This commitment stems from the recognition that robust data governance is crucial for harmonizing data activities across an organization, ensuring data quality, compliance, and security. According to recent insights, around 60% of corporate leaders have placed a high priority on improving their data governance frameworks. This focus is particularly evident among chief data officers (CDOs) who see data governance as a foundational element that precedes other data initiatives like artificial intelligence (AI).
Interestingly, data governance is outpacing AI in terms of priority among data platform and security practitioners. This trend highlights the growing awareness that without effective data governance, the potential benefits of AI and other advanced data technologies cannot be fully realized. The return on investment (ROI) from implementing strong data governance practices is a significant motivator for this focus. Organizations are increasingly recognizing that data governance is not just a regulatory requirement but a strategic asset that can drive business value and efficiency.
Dr. Peter Aiken, a recognized authority in Data Management and the president of DAMA International, highlights a critical issue in the realm of data governance: the substantial cost associated with fixing poor data governance practices. Research from Gartner reveals that poor data quality costs organizations an average of $15 million annually. Similarly, IBM found that businesses in the U.S. lose a staggering $3.1 trillion each year due to inadequate data governance. These significant losses highlight the missed opportunities to invest in innovative data governance initiatives
This figure underscores the significant financial burden that ineffective data governance can place on organizations. Instead of investing in cutting-edge technologies like AI, machine learning, or advanced analytics, companies often find themselves dedicating a large portion of their resources to rectifying issues stemming from inadequate data management. This not only limits their ability to innovate but also hinders their overall operational efficiency.
Recent developments in data governance for 2024 reflect the increasing importance of managing and protecting data as organizations navigate a complex landscape of data-driven innovation and regulatory requirements.
Key Trends
These trends highlight the evolving role of data governance as not just a compliance necessity, but a strategic asset that enhances the overall data management strategy of organizations.
Data Governance at a Bank – A Usage Scenario
Data governance in the finance industry is crucial for ensuring the accuracy, security, and compliance of financial data.
Here’s an example of how data governance can be conceptualized in a Bank:
1. Define Data Governance Objectives
Objective
Establish goals and objectives for data governance.
Actions
Identify the bank’s primary data governance goals keeping an eye on regulatory compliance, data quality and risk management. Create a data governance objective that outlines data management principles, data quality standards, and compliance requirements.
2. Establish Data Governance Framework
Objective
The Bank must consider developing a framework that includes policies, standards, and roles to ensure consistency and compliance.
Actions
- Define policies for data usage, security, access, retention, and deletion.
- Set data standards (e.g., naming conventions, data formats) and quality requirements.
3. Form a Data Governance Team
Objective
Assemble a cross-functional team (from various departments) responsible for data governance.
Actions
- Engage stakeholders from various functions (e.g., compliance, IT, operations) to align objectives.
- Include roles such as Data Stewards, Data Custodians, Data Architects, and Compliance Officers.
- Define responsibilities for each role, including data quality monitoring, access management, and issue resolution. Establish a RACI (Responsible, Accountable, Consulted, Informed) matrix to clarify roles.
4. Develop Data Governance Policies
Objective
Create and document policies for data management.
Actions
Define data ownership, data access controls, data retention policies, and data privacy measures.
5. Implement Data Classification
Objective
Categorize data based on sensitivity and importance.
Actions
Classify data into categories such as public, internal, confidential, and restricted. Implement access controls based on these classifications.
6. Data Quality Management
Objective
Ensure the accuracy, consistency, and completeness of financial data.
Actions
Implement data validation processes, regular data quality audits, and data cleansing procedures to correct any discrepancies.
7. Compliance and Risk Management
Objective
Ensure adherence to regulatory requirements and manage data-related risks.
Actions
Monitor compliance with regulations such as GDPR, CCPA, and financial regulations (e.g., Basel III). Conduct risk assessments and implement mitigation strategies.
8. Data Integration and Architecture supporting Data Governance
Objective
Ensure data governance as data integration happens across systems.
Actions
Design a data architecture incorporating data governance principles, that support data integration from various sources, including transactional systems, data warehouses, and external data feeds.
9. Data Security Measures
Objective
Protect sensitive financial data from unauthorized access and breaches.
Actions
Implement RBAC (Role Based Access Control), encryption, multi-factor authentication, and intrusion detection systems. Regularly update security protocols and conduct security training for staff.
10. Monitor and Review
Objective
Continuously assess the effectiveness of data governance practices.
Actions
Establish metrics and KPIs to monitor data governance performance. Regularly review and update data governance policies based on changes in regulations and business needs.
11. Stakeholder Communication
Objective
Ensure all stakeholders are informed and engaged.
Actions
Communicate data governance policies and procedures to employees, customers, and partners. Provide training and support to ensure adherence.
By implementing these steps, a financial organization like a bank can effectively manage its data, ensuring it is accurate, secure, and compliant with regulatory standards. This approach not only enhances operational efficiency but also builds trust with customers and regulators.
Reference:
- Michelle Knight on January 30, 2024, https://www.dataversity.net/data-governance-trends-in-2024/